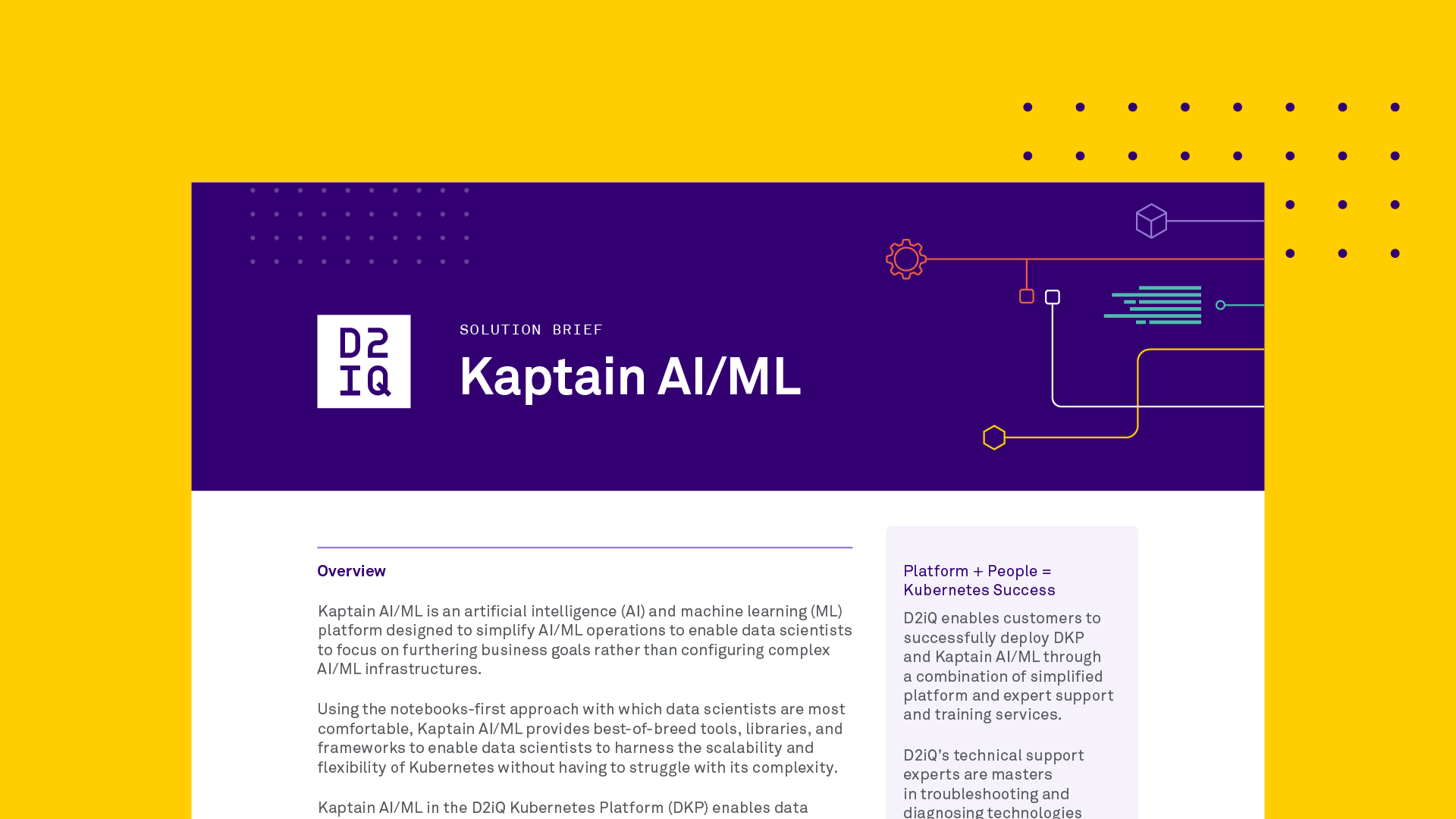
Free Download: Kaptain AI/ML: Simplified AI/ML Operations
Kaptain AI/ML is an artificial intelligence (AI) and machine learning (ML) platform designed to simplify AI/ML operations to enable data scientists to focus on furthering business goals rather than configuring complex AI/ML infrastructures.